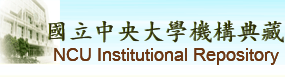 |
English
|
正體中文
|
简体中文
|
全文筆數/總筆數 : 80990/80990 (100%)
造訪人次 : 42646205
線上人數 : 973
|
|
|
資料載入中.....
|
請使用永久網址來引用或連結此文件:
http://ir.lib.ncu.edu.tw/handle/987654321/84390
|
題名: | 以深度摺含神經網路重建擴散光學影像研究;Diffuse Optical Imaging using Deep Convolutional Neural Networks |
作者: | 代安語;Yuliansyah, Diannata Rahman |
貢獻者: | 機械工程學系 |
關鍵詞: | 擴散光學成像;深度卷積神經網絡;Tikhonov正則化;Diffuse Optical Imaging;deep convolutional neural networks;Tikhonov regularization |
日期: | 2020-08-24 |
上傳時間: | 2020-09-02 19:15:10 (UTC+8) |
出版者: | 國立中央大學 |
摘要: | 本研究的目的是開發深度學習算法,以替代現有的Tikhonov正則化方法。在本研究中,我們開發了一個用於擴散光學成像的深度卷積神經網絡模型。我們準備的訓練數據集由10000個樣本組成,其中有不同命名的仿體案例。這些仿體案例的參數已經根據不同的參數設定。對於每個樣本,輸入數據的形式是16×15×2浮點值(16個光源與檢測器位置),即對數振幅和對數相位值。輸出數據的形式是大小為64×64的矩形網格,每個網格的吸收和散射係數。這些值是從3169個節點的原始數據內插出來的。對於測試數據集,從實驗數據中共選取了10個樣本。該模型架構基於多種方法。我們使用了從傳感器域到圖像域轉換的方法。我們還使用了編碼器的概念,就是學習輸入的壓縮表示。將輸入的壓縮和轉換到圖像域後,我們使用U-net與跳過連接來提取特徵,得到對比度圖像。將對比度圖像與背景係數相乘得到輸出圖像。 在訓練過程中,我們使用自定義的損失函數,它是對比圖像和背景係數輸出的加權MSE之和。我們使用Adam優化器,β1=0.5。我們使用的學習率為0.0002,批次大小為32。該模型共創建了6,588,608個可訓練參數,並在21.6小時內訓練了200個迭代。同時,對於訓練數據集中的每個樣本,Tikhonov正則化方法的平均計算為154秒。 僅經過幾次迭代,訓練損失就會迅速下降,因此深度學習的體系結構被認為是理想的。 我們選擇使用第七個時期的權重,因為它證明這可以使模型能夠預測未知的實驗數據。 需要進行進一步的概括,以確保模型不會過擬合併提高性能。 從結果可以得出結論,由於該模型成功地定位了夾雜物,因此該模型可以替代Tikhonov正則化方法。 關鍵字:擴散光學成像,深度卷積神經網絡,Tikhonov正則化 ;The purpose of this study is to develop deep learning algorithms as an alternative to the existing Tikhonov regularization method. In this study, a deep convolutional neural network model for diffuse optical imaging has been developed. We prepare the training dataset that consists of 10000 samples with different designations of phantom cases. The parameters of these phantom cases had been specified based on various properties. For each sample, the input data are in the form of 16×15×2 floating-point values (16 source/detector locations), which are log amplitude and log phase values. The output data are in the form of a rectangular grid of size 64×64, each for both absorption and scattering coefficients. These values have been interpolated from the original data of 3169 nodes. For the test dataset, there are a total of 10 chosen samples from experimental data. The model architecture is based on multiple ideas. We use the idea of transformation from sensor-domain to image-domain. We also use the concept of an encoder, which is to learn a compressed representation of the inputs. After the compression and transformation of the inputs to image-domain, we use U-net with skip connections to extract the features and obtain the contrast image. The output images are obtained by multiplying the contrast images with the background coefficients. For the training process, we use custom loss function which is the sum of the weighted MSE of contrast image and background coefficient outputs. We use Adam optimizer with β1 = 0.5. We use a learning rate of 0.0002 and a batch size of 32. The model has been created with a total of 6,588,608 trainable parameters and trained in 21.6 hours for 200 epochs. Meanwhile, the average computation for the Tikhonov regularization method is 154 seconds for each sample in the training dataset. The training loss drops quickly after only a few iterations, hence the architecture of deep learning is considered ideal. We choose to use the weights resulted from the seventh epoch, as it proves that this can make the model to be able to predict the unseen experimental data. A further generalization is needed so that the model doesn’t overfit and the performance can be enhanced. From the results, it is concluded that the proposed model is feasible as an alternative to the Tikhonov regularization method, as the proposed model succeed to localize the inclusions. |
顯示於類別: | [機械工程研究所] 博碩士論文
|
文件中的檔案:
檔案 |
描述 |
大小 | 格式 | 瀏覽次數 |
index.html | | 0Kb | HTML | 171 | 檢視/開啟 |
|
在NCUIR中所有的資料項目都受到原著作權保護.
|
::: Copyright National Central University. | 國立中央大學圖書館版權所有 | 收藏本站 | 設為首頁 | 最佳瀏覽畫面: 1024*768 | 建站日期:8-24-2009 :::