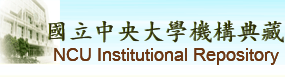 |
English
|
正體中文
|
简体中文
|
全文笔数/总笔数 : 78852/78852 (100%)
造访人次 : 35338822
在线人数 : 333
|
|
|
数据加载中.....
|
jsp.display-item.identifier=請使用永久網址來引用或連結此文件:
http://ir.lib.ncu.edu.tw/handle/987654321/84871
|
题名: | 基於LSTM方法於建置預診斷與健康管理模型—以塗佈機為例;Developing a Prognostics and Health Management Model Based on LSTM Approach – A Case Study of Coating Machine |
作者: | 陳嘉瑩;Chen, Jia-Ying |
贡献者: | 工業管理研究所 |
关键词: | 預診斷與健康管理;異常檢測;深度學習;長短期記憶網路;自動編碼器;Prognostics and Health Management;Anomaly Detection;Deep Learning;Long Short-Term Memory Network;Autoencoder |
日期: | 2021-01-14 |
上传时间: | 2021-03-18 16:41:46 (UTC+8) |
出版者: | 國立中央大學 |
摘要: | 在工業4.0 的浪潮下,全球製造業以「智慧製造」為主軸,朝向「智動化」的目標發展,精準地制定維護策略有助於提高生產效率及產品品質,成為智慧製造的關鍵議題之一。物聯網(Internet of Things, IoT)、感測器(Sensor)、數據分析(Big Data)、雲端運算(Cloud Computing)及人工智慧(Artificial Intelligence, AI)等日臻成熟的先進技術,促使預測性維護得以實現。許多機器設備都配置了感測器並結合物聯網收集大量的數據,然而,更重要的任務是從數據資料中挖掘出可利用的資訊,並做出更好的決策,才能使資料被物盡其用,發揮最大的價值。 本研究欲使用A公司所提供之塗佈機,以預診斷與健康管理(Prognostics and Health Management, PHM)為主題改善該公司原有的預防性維護策略,建立塗佈機的預測模型。透過深度學習演算法中的長短期記憶(Long Short-Term Memory, LSTM)神經網路結合自動編碼器(Autoencoder)作為建置模型的方法,從塗佈機運作的歷史數據中分析後獲得規律,找出判斷異常狀況的徵兆以對機台進行異常檢測與條件監控,並建立評估機台健康狀況之指標。預測結果與實際狀況相比,可以於異常前10秒檢測到徵兆,並與實際張力異常紀錄相比提早20秒判斷出異常,加總之下爭取到30秒的反應時間,有助於人員能在停機前做出適切的維護決策,確保生產線的正常運作,進而達到預測維護之目的。;Under the trend of Industry 4.0, manufacturing aims at achieving “Intelligent Manufacturing”. Formulating the maintenance strategy accurately contributes to improving the production efficiency and product quality. Recently, significant attention has been paid to predictive maintenance. The rapid development of science and technology, such as the IoT, sensor, cloud computing, and AI has paved the way for the predictive maintenance. Most of the machines have assembled sensors connecting to IoT to acquire data. To make the best of the data, companies should focus on how to convert the data into valuable information to support maintenance decision making. This study uses the production dataset of the coating machine provided by Company A, intending to conduct a health assessment while establishing a prediction model based on the Prognostics and Health Management framework, thereby improving the company′s original preventive maintenance strategy. Combing Autoencoder with LSTM neural network to construct a predictive model, extracting the characteristics through the historical data transferred from the coating machine for condition monitoring and finding out the signs of an anomaly when there is an abnormal situation. Then, build up the health index to do a health assessment for the coating machine. The results show that the predicted model can detect 30 seconds in total earlier than the actual abnormal record, thereby enabling personnel to make appropriate maintenance decisions within the reaction time before the breakdown, maximizing the uptime of equipment while avoiding disruption to production and reaching the predictive maintenance. |
显示于类别: | [工業管理研究所 ] 博碩士論文
|
文件中的档案:
档案 |
描述 |
大小 | 格式 | 浏览次数 |
index.html | | 0Kb | HTML | 178 | 检视/开启 |
|
在NCUIR中所有的数据项都受到原著作权保护.
|
::: Copyright National Central University. | 國立中央大學圖書館版權所有 | 收藏本站 | 設為首頁 | 最佳瀏覽畫面: 1024*768 | 建站日期:8-24-2009 :::