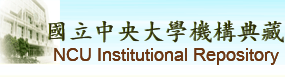 |
English
|
正體中文
|
简体中文
|
全文筆數/總筆數 : 80990/80990 (100%)
造訪人次 : 42716959
線上人數 : 1553
|
|
|
資料載入中.....
|
請使用永久網址來引用或連結此文件:
http://ir.lib.ncu.edu.tw/handle/987654321/86457
|
題名: | 應用長短期記憶網路模型於學習成效的早期預測以進行及時干預;Applying LSTM on Early Prediction for Timely Intervention |
作者: | 陳昱蓉;Chen, Yu-Rong |
貢獻者: | 資訊工程學系 |
關鍵詞: | 學習分析;早期預測;長短期記憶網路;及時干預;learning analytics;early prediction;Long-Short-Term-Memory;timely intervention |
日期: | 2021-07-12 |
上傳時間: | 2021-12-07 12:51:29 (UTC+8) |
出版者: | 國立中央大學 |
摘要: | 本研究透過蒐集學生於線上學習環境的學習軌跡來預測學生的學習成效。為了驗證時序性特徵對於學習預測是否能提升效能,我們提出能夠接受時序性資料的voting-based LSTM model,它是一個基於LSTM(Long Short-term Memory)架構的模型,並與傳統的學習預測方法SVM(Support Vector Machine)進行效能比較,並依據預測結果設計了干預措施於實驗組班級進行,最後比較實驗組班級與控制組班級在干預前後的周學習分數及有風險學生人數變化,來探討干預措施對於學生的學習成效之影響。此外,由於參與課程中的學生來自各個不同的科系,主要可以分為文管學院及理工學院二種不同的類型,而不同類型的學生在學習背景及邏輯思考方式上可能存在差異,我們想從蒐集到的數據探討學生的學習方式是否會由於不同的學習背景而有所差異。 研究結果顯示加入時間相關的資訊的確有助於提升學習預測的效能,且實施了干預措施的實驗組班級除了在周學習分數有顯著的提升外,在有風險學生的人數上也有大大的改善,也就是說干預措施對於學生的學習成效是有幫助的。此外,根據分析線上學習平台的學習歷程,也發現了不同學習背景的學生的確存在學習方式上的差異。;In our research, we collected the learning logs from an online learning environment to predict students’ learning outcomes. To verify whether temporal features can improve the performance of learning prediction, we propose a voting-based LSTM model, which is an architecture based on LSTM(Long Short-term Memory), that can accept temporal information as inputs to compare the performance with SVM (Support Vector Machine), which is a traditional learning prediction method. Based on the results of prediction, we designed an intervention measure which was carried out in the experimental group. And we compared the weekly learning scores of the experimental group and the control group and the changes in the number of at-risk students before the intervention and after the intervention separately to explore the impact of intervention measures on students′ learning outcomes. Additionally, since the students participating in this course come from a lot of different departments, and they could be divided into two main types, one is namely "Liberal Arts and Management college", and the other one is "Science and Technology college". We thought that students from these two different types might have differences in their learning backgrounds and logical thinking. Thus, we wanted to know whether different learning backgrounds result that students have different learning styles. The results show that adding temporal features does have a benefit in improving the performance of learning prediction. And we compared the experimental group and the control group in the students′ weekly learning scores and the number of at-risk students, which was from the result of prediction. We found that after the intervention measures, not only the weekly learning scores significantly increased, but also the ratio of at-risk students was lower in the experimental group. In other words, the intervention measure we designed is helpful to improve student′s learning outcomes. On the other hand, according to the analysis of the learning logs of the online learning platform, it also reveals that there do exist learning differences due to the differences of their learning backgrounds. |
顯示於類別: | [資訊工程研究所] 博碩士論文
|
文件中的檔案:
檔案 |
描述 |
大小 | 格式 | 瀏覽次數 |
index.html | | 0Kb | HTML | 58 | 檢視/開啟 |
|
在NCUIR中所有的資料項目都受到原著作權保護.
|
::: Copyright National Central University. | 國立中央大學圖書館版權所有 | 收藏本站 | 設為首頁 | 最佳瀏覽畫面: 1024*768 | 建站日期:8-24-2009 :::