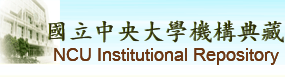 |
English
|
正體中文
|
简体中文
|
全文筆數/總筆數 : 80990/80990 (100%)
造訪人次 : 42700473
線上人數 : 1520
|
|
|
資料載入中.....
|
請使用永久網址來引用或連結此文件:
http://ir.lib.ncu.edu.tw/handle/987654321/86756
|
題名: | 基於深度學習之冠狀動脈分割及其應用;The Development of Deep Learning-based Coronary Artery Segmentation Methods and its Applications |
作者: | 游子謙;Yu, Tzu-Chien |
貢獻者: | 資訊工程學系 |
關鍵詞: | 冠狀動脈;深度學習;醫學影像;影像處理;電腦視覺;Coronary Artery;Deep Learning;Medical Image Processing;Image Processing;Computer Vision |
日期: | 2021-08-25 |
上傳時間: | 2021-12-07 13:11:18 (UTC+8) |
出版者: | 國立中央大學 |
摘要: | 根據台灣衛生福利部統計處統計,心臟疾病長年位居台灣前三大死因,其中冠狀動脈心臟病又是十分常見的心臟疾病之一。目前在冠狀動脈檢查時,經常使用非侵入性的電腦斷層掃描,透過於電腦斷層影像中標註冠狀動脈位置,可以進行後續的血管狹窄度、鈣化位置的分析,然而以人工方式進行冠狀動脈血管標註非常花費時間,因此如何自動化流程,以減少過程中所耗費的人力資源,便顯得十分重要。
除此之外,電腦斷層掃描中使用的顯影劑通常含有碘的成分,對於部分受檢者可能產生過敏或對其腎臟造成傷害,因此較不適合使用顯影劑,然而無顯影劑增強之電腦斷層掃描影像對於血管的對比度較低,顯著地增加了冠狀動脈血管的標註難度,因此如何運用無顯影劑增強之電腦斷層影像進行冠狀動脈分割,也是具有挑戰性的問題。
本研究利用深度學習技術,使用有顯影劑增強之電腦斷層影像,訓練能夠自動地從電腦斷層影像中分割冠狀動脈的模型,並且利用分割之結果開發應用, 進行鈣化位置以及血管狹窄度的分析。此外本研究也使用無顯影劑增強之電腦斷層影像進行實驗,使用深度學習技術實現一個資料擴增模型,將有顯影劑增強之電腦斷層掃描影像轉換為虛擬的無顯影劑增強之電腦斷層影像,並將產生的影像利用於輔助訓練以無顯影劑增強之電腦斷層影像進行冠狀動脈分割的模型。
實驗結果顯示,本研究能夠利用有顯影劑增強之電腦斷層影像有效的分割冠狀動脈,對於測試資料以7-fold交叉驗證能夠達到Dice Coefficient 0.7868的結果,並能夠以此分割結果進行鈣化位置以及血管狹窄度分析,給予醫師在診斷上的輔助。而在無顯影劑增強之電腦斷層影像實驗中,透過利用本研究的資料擴增方式產生的訓練資料輔助模型訓練,對於測試資料以5-fold交叉驗證能夠從Dice Coefficient 0.5158增加到0.5674,顯示了該資料擴增方法的有效性。 ;According to the report from the Statistics Department of Taiwan’s Ministry of Health and Welfare, heart disease has been among the top three causes of death in Taiwan for many years. Among them, coronary artery disease is one of the most common heart diseases. Currently, computed tomography (CT) is often used in coronary artery examinations. By extracting the coronary arteries in the CT image, the subsequent analysis of vascular stenosis and calcification can be carried out. However, manual labeling of coronary arteries is a time-consuming task. Therefore, how to automate the process to reduce the human resources consumed in the process is very important.
In addition, the contrast agent used in computed tomography usually contains iodine. For some subjects, the contrast agent may cause allergies or damage their kidney function, so those subjects are unsuitable for the contrast agent. However, the contrast of CT images without contrast enhancement to vessels is very low, and it significantly increases the difficulty of labeling coronary arteries. Therefore, how to use CT images without contrast enhancement for coronary artery segmentation is also a challenging problem.
This research uses CT images with contrast enhancement to train a model that can automatically segment coronary arteries from CT images and use the results of segmentation to develop applications that perform analysis of calcification location and vascular stenosis. In addition, this research also used CT images without contrast enhancement for experiments. This research uses deep learning techniques to implement a data augmentation model to convert the CT image with contrast enhancement into a virtual CT image without contrast enhancement. The generated virtual CT images are then used to assist in training a model of coronary artery segmentation with CT images without contrast enhancement.
Experimental results show that this research′s deep learning model can effectively extract coronary arteries from CT images with contrast enhancement. For the test CT images with contrast enhancement, the result of Dice Coefficient 0.7868 can be achieved. The segmentation results can be used to analyze calcification and vascular stenosis, and assist physicians in diagnosis. For the CT images without contrast enhancement experiment, the test result can be increased from Dice Coefficient 0.5158 to 0.5674 by using the training data generated by the data augmentation model of this study. The results show the effectiveness of the data augmentation model. |
顯示於類別: | [資訊工程研究所] 博碩士論文
|
文件中的檔案:
檔案 |
描述 |
大小 | 格式 | 瀏覽次數 |
index.html | | 0Kb | HTML | 65 | 檢視/開啟 |
|
在NCUIR中所有的資料項目都受到原著作權保護.
|
::: Copyright National Central University. | 國立中央大學圖書館版權所有 | 收藏本站 | 設為首頁 | 最佳瀏覽畫面: 1024*768 | 建站日期:8-24-2009 :::