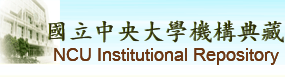 |
English
|
正體中文
|
简体中文
|
全文筆數/總筆數 : 80990/80990 (100%)
造訪人次 : 42686533
線上人數 : 1539
|
|
|
資料載入中.....
|
請使用永久網址來引用或連結此文件:
http://ir.lib.ncu.edu.tw/handle/987654321/88331
|
題名: | 基於台語與華語之語碼混合資料集與翻譯模型;Hokkien-Mandarin Code-Mixing Dataset and Neural Machine Translation |
作者: | 呂昕恩;Lu, Sin-En |
貢獻者: | 資訊工程學系在職專班 |
關鍵詞: | 語碼混合;機器翻譯;損失函數重構;低資源語言;Code-Mixing;Neural Machine Translation;Loss Function Reconstruction;Low Resource;WordNet |
日期: | 2022-01-21 |
上傳時間: | 2022-07-13 22:46:58 (UTC+8) |
出版者: | 國立中央大學 |
摘要: | 台語與中文語碼混合在台灣是一個常見的口語現象,然而台灣遲至 21 世紀才開始建立官方書寫系統。缺少官方書寫系統,不僅代表著我們在 NLP 領域面臨資源不足的問題,導致我們在方言代碼混合任務上難以取得突破性研究,更意味著我們面臨著語言傳承的困難。基於上述問題,本研究將從簡要介紹台語的歷史以及台灣語碼混合現象著手,討論台灣語碼混合的語言比例組成與文法結構,建立基於台文字的台語語華語之語碼混合資料集,並介紹可應用於台文的現有斷詞工具。 同時我們將在本研究介紹台語語言模型的訓練方法,同時使用我們提出的資料集,利用 XLM 開發台語語碼混合翻譯模型。
為適用於語碼混合的情境,我們提出自動化語言標注(DLI)機制,並使用遷移學習提升翻譯模型表現。 最後我們根據交叉熵(Cross-entropy, CE)的問題,提出三種利用詞彙詞相似度來重構損失函數。我們提出 WBI 機制,解決詞彙資訊與字符集預訓練模型不相容的問題,並引入 WordNet 知識在模型中。與標準 CE 相比,在單語和語碼混資料集的實驗結果表明,我們的最佳損失函數在單語和 CM 在 BLEU 上,分別進步 2.42分(62.11 到 64.53)和 0.7(62.86 到 63.56)分。我們的實驗證明即使是使用基於字符訓練的語言模型,我們可以將辭彙的資訊攜帶到下游任務中。;Code-mixing is a complicated task in Natural Language Processing (NLP), especially for mixed languages are dialects. In Taiwan, code-mixing is a common phenomenon. The most popular code-mixed language pair is Hokkien and Mandarin. However, there is a lack of resources in Hokkien. Therefore, we proposed a Hokkien-Mandarin code-mixing dataset and offered the efficient Hokkien word segment method through an open-source toolkit. These could overcome the morphology issue under the Sino-Tibetan family. We modify an XLM model (cross-lingual language model) with the dynamic language identify (DLI) mechanism and use transfer learning to train our proposed dataset on translation tasks. We found that by applying language knowledge, rules and offering the language tags, the model achieves good performance on code-mixing data translation results and maintains the quality of monolingual translation.
Recently, most neural machine translation models (NMT) use cross-entropy as the loss function, including XLM model. However, standard cross-entropy penalizes the model when it fails to generate ground truth answers, eliminating the opportunity to consider other possibilities. It can cause problems with \textit{overcorrection} or \textit{over-confident}. Some solutions to reconstruct the loss function using word similarity have been proposed. But these solutions are not suitable for Chinese because most Chinese models are pre-trained at the character level. In this work, we propose a simple but effective method, Word Boundary Insertion (WBI), to address the inconsistency between word-level and character-level by reconstructing the loss function of Chinese NMT models. WBI considers word similarity without modifying or retraining a new language model. We propose three modified loss functions for use with XLM, and the calculation of these loss functions also refers to the WordNet. Compared with the standard cross-entropy, experimental results on both monolingual and code-mixing (code-mixing) Hokkien-Chinese datasets show that our best loss function achieves BLEU score improvements of 2.42 (62.11 to 64.53) and 0.7 (62.86 to 63.56) on monolingual and code-mixing data, respectively. |
顯示於類別: | [資訊工程學系碩士在職專班 ] 博碩士論文
|
文件中的檔案:
檔案 |
描述 |
大小 | 格式 | 瀏覽次數 |
index.html | | 0Kb | HTML | 121 | 檢視/開啟 |
|
在NCUIR中所有的資料項目都受到原著作權保護.
|
::: Copyright National Central University. | 國立中央大學圖書館版權所有 | 收藏本站 | 設為首頁 | 最佳瀏覽畫面: 1024*768 | 建站日期:8-24-2009 :::