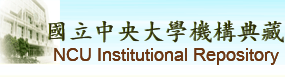 |
English
|
正體中文
|
简体中文
|
全文筆數/總筆數 : 80990/80990 (100%)
造訪人次 : 42678510
線上人數 : 1360
|
|
|
資料載入中.....
|
請使用永久網址來引用或連結此文件:
http://ir.lib.ncu.edu.tw/handle/987654321/89376
|
題名: | 基於深度學習的反射震測速度分析;Analysis of Reflection Seismic Velocity Based on Deep Learning |
作者: | 林東璋;Lin, Dong-Jhang |
貢獻者: | 地球科學學系 |
關鍵詞: | 反射震測法;速度分析;深度學習;seismic reflection method;velocity analysis;deep learning |
日期: | 2022-08-25 |
上傳時間: | 2022-10-04 11:12:32 (UTC+8) |
出版者: | 國立中央大學 |
摘要: | 反射震測法常用於探測地下構造並建構地下速度模型,在反射震測資料處理流程中,最關鍵的步驟之一為速度分析。速度分析的品質好壞將直接關係到最終地下構造的成像品質。傳統的速度分析方法為先計算出波線資料的速度譜(velocity semblance spectrum)後,經由人工判讀點選速度譜相對聚焦處以得知各個地層相對應的反射波走時及地層速度。然而,反射震測測線往往動輒數十至數百公里長,若完全經由人工分析將曠日費時。此外,目前國際學界雖已積極利用深度學習方法開發人工智慧方式挑選震測地層速度,但尚無完全克服在受雜訊影響下開發出可信賴之自動化速度分析方法。本研究旨在借助深度學習方法,開發快速有效的自動化速度分析方法,以期在不影響最終成像品質的狀況下,加速反射震測資料處理流程,以利研究者能更快速地進行後續震測地層分析。 本研究將反射震測速度分析問題視為圖像辨識中的語意分割(semantic segmentation)問題。輸入資料為速度譜,運用加入注意力門(Attention Gates, AGs)的U-Net神經網路模型對速度譜中的各個資料點進行分類。另外,因無法得知真實地下構造,野外資料皆為無標註資料(unlabeled data)。因此,本研究使用有限差分數值模擬之震測資料(synthetic seismic data)進行訓練,並在部分資料中加入隨機雜訊以貼近真實情況。經訓練後於測試資料集可得精確率(precision)、召回率(recall)及F1值分別為0.947、0.590及0.727,經過訓練後的網路可辨別真實地層訊號與複反射訊號造成的速度譜振幅高點。於1995年TAICRUST計畫所搜集之EW9509-1測線實測結果可發現,本研究提出之模型可於沉積盆地(沖繩海槽及弧前盆地)之淺層地層有良好的速度預測結果,而於缺乏反射面之火山島弧區域(琉球島弧)及深部地層有較差的預測結果,此可能與本研究模型未考慮波浪雜訊及模型最大速度僅止於每秒五公里有關,未來可進一步改善以更適合現地震測資料使用。;The seismic reflection method is often used to detect underground structures and construct subterranean velocity models. In the workflow of reflection seismic data processing, one of the most critical steps is velocity analysis. The quality of velocity analysis will directly affect the imaging quality of the final underground structure. The traditional velocity analysis method is to calculate the velocity semblance spectrum from original seismic data, manually interpret and pick the relative local semblance spectrum amplitude high and derives the corresponding reflected wave travel time and velocity of each horizon. However, due to tens to hundreds of kilometers long seismic profiles, manual picking velocity is time-consuming and has a less spatial resolution. This study aims to develop a fast and effective automatic velocity analysis method by using deep learning techniques, producing a high-quality velocity model and accelerating the reflection seismic data processing workflow. In this study, we considered velocity analysis problem as a semantic segmentation problem. The input data is the velocity semblance spectrum, and each data point in the input is classified using the U-Net architecture with Attention Gates (AGs). In addition, due to underground structures being unknown, the field seismic data are unlabeled. Thus the training data of this study is composed of synthetic seismic data and data with random noise from finite difference simulation. After training, results show precision of 0.947, recall of 0.590 and F1 score of 0.727, respectively. Our model can recognize velocity semblance spectrum amplitude highs, picking accurate velocity and avoiding water column multiples. In addition to a long-offset multichannel seismic profile EW9509-1 collected by TAICRUST project in 1995, our model behaves well in sedimentary basins but behaves poorly in arc volcanism area and deep sedimentary basins. This is probably because our training dataset did not consider deep (limited to 5 km/s in velocity) or complex crustal structures and did not include water column noise generated from tide and artificial sources, which can be improved in the future. |
顯示於類別: | [地球物理研究所] 博碩士論文
|
文件中的檔案:
檔案 |
描述 |
大小 | 格式 | 瀏覽次數 |
index.html | | 0Kb | HTML | 108 | 檢視/開啟 |
|
在NCUIR中所有的資料項目都受到原著作權保護.
|
::: Copyright National Central University. | 國立中央大學圖書館版權所有 | 收藏本站 | 設為首頁 | 最佳瀏覽畫面: 1024*768 | 建站日期:8-24-2009 :::