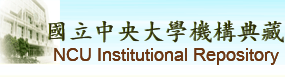 |
English
|
正體中文
|
简体中文
|
全文筆數/總筆數 : 80990/80990 (100%)
造訪人次 : 42717415
線上人數 : 1531
|
|
|
資料載入中.....
|
請使用永久網址來引用或連結此文件:
http://ir.lib.ncu.edu.tw/handle/987654321/89809
|
題名: | 深度學習菁英基因演算法之線切割放電加工多目標參數最佳化;Wire Electrical Discharge Machining Multi-objective Parameter Optimization with Deep Learning Elitist Genetic Algorithm |
作者: | 陳思翰;Chen, Si-Han |
貢獻者: | 資訊工程學系 |
關鍵詞: | 線切割放電加工;代理模型;非超越排序基因演算法;菁英基因演算法;遷移學習;加工參數最佳化;wire electrical discharge machining;surrogate model;non-dominated sorting genetic algorithm;elitist genetic algorithms;transfer learning;machining parameter optimization |
日期: | 2022-07-26 |
上傳時間: | 2022-10-04 12:00:38 (UTC+8) |
出版者: | 國立中央大學 |
摘要: | 本論文提出線切割放電加工(wire electrical discharge machining, WEDM)多目標參數最佳化方法(multi-objective parameter optimization, MOPO),可以針對指定的產品品質要求,自動產出最佳加工參數組合建議。其基本概念為在多目標演化演算法框架(multi-objective evolutionary algorithm framework)中,以深度神經網路(deep neural network, DNN)為基礎,透過收集「線切割放電機-A」的大量加工資料建構資料驅動代理模型(data-driven surrogate model),並採用第二代非超越排序基因演算法(nondominated sorting genetic algorithm II, NSGA-II),即菁英基因演算法(elitist genetic algorithm),輸出逼近多目標最佳化之加工參數組合建議,完成建構線切割放電加工多目標參數最佳化方法(WEDM-MOPO)的目標。另外,WEDM-MOPO也透過權重凍結(weight freezing)之遷移學習(transfer learning)概念,使用小量「線切割放電機-B」的加工資料將「線切割放電機-A」的深度神經網路代理模型移轉為「線切割放電機-B」的深度神經網路代理模型,以擴增WEDM-MOPO方法的適用範圍。本論文使用實際WEDM加工資料進行實驗,實驗結果顯示「線切割放電機-A」以及「線切割放電機-B」的深度神經網路代理模型均可準確預測線切割放電機的產品品質,在加工速度(machining speed or feed rate)及表面粗糙度(surface roughness)方面,達到平均絕對誤差百分比(mean absolute percentage error, MAPE)為5%以下的目標。另外,在工件精度(workpiece precision)方面,則達到平均絕對誤差(mean absolute error, MAE)為3 μm以下的目標。藉由深度神經網路代理模型的精準預測,WEDM-MOPO因而可以產出良好的最佳加工參數組合建議。另外,再根據對應的產品品質預測進一步選擇幾組特定的最佳加工參數組合並應用於實際的放電加工。其真實產品品質顯示WEDM-MOPO建議的最佳化加工參數組合確實可以滿足指定的產品品質要求。;In this thesis, a wire electrical discharge machining multi-objective parameter optimization (WEDM-MOPO) method is proposed, which can automatically generate the best machining parameter combination recommendations for specified machining quality requirements. The basic concept is to first train a deep neural network (DNN) as a data-driven surrogate model of the multi-objective evolutionary algorithm framework, by a large amount of processing data collected from a "WEDM-A" machine. Afterwards, the method uses the nondominated sorting genetic algorithm II (NSGA-II), that is the elitist genetic algorithm, to output machining parameter combinations for the specified machining quality. In addition, WEDM-MOPO also uses a small amount of processing data collected from a "WEDM-B" machine to transfer the DNN model for "WEDM-A" to be the DNN model for "WEDM-B" through the transfer learning concept of weight freezing. The surrogate DNN model transferring can expand the scope of WEDM-MOPO applications. Extensive experiments using practical WEDM processing data are conducted. Experimental results show that the DNN surrogate models of "WEDM-A" and "WEDM-B" can accurately predict the machining quality of WEDM. In terms of machining speed and surface roughness, the models have the mean absolute percentage error (MAPE) that are less than 5%. In addition, in terms of workpiece precision , the model have the mean absolute error (MAE) that are less than 3 μm. With the accurate prediction of the DNN surrogate models, WEDM-MOPO can generate good recommendations of optimal machining parameter combinations. Recommended machining parameters are selected according to their associated production quality prediction and applied to practical WEDM machining. It is shown that the machining parameters recommended by WEDM-MOPO indeed meet the specified machining quality requirements. |
顯示於類別: | [資訊工程研究所] 博碩士論文
|
文件中的檔案:
檔案 |
描述 |
大小 | 格式 | 瀏覽次數 |
index.html | | 0Kb | HTML | 61 | 檢視/開啟 |
|
在NCUIR中所有的資料項目都受到原著作權保護.
|
::: Copyright National Central University. | 國立中央大學圖書館版權所有 | 收藏本站 | 設為首頁 | 最佳瀏覽畫面: 1024*768 | 建站日期:8-24-2009 :::