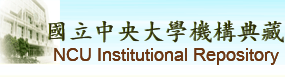 |
English
|
正體中文
|
简体中文
|
全文筆數/總筆數 : 80990/80990 (100%)
造訪人次 : 42686443
線上人數 : 1422
|
|
|
資料載入中.....
|
請使用永久網址來引用或連結此文件:
http://ir.lib.ncu.edu.tw/handle/987654321/93220
|
題名: | 深度學習唇語辨識之研究;A Study on Lip Reading Recognition using Deep Learning |
作者: | 魏湘凌;Wei, Hsiang-Ling |
貢獻者: | 資訊管理學系在職專班 |
關鍵詞: | 深度學習;唇語辨識 |
日期: | 2023-07-25 |
上傳時間: | 2024-09-19 16:49:20 (UTC+8) |
出版者: | 國立中央大學 |
摘要: | 近年來,深度學習已成為人工智慧領域的一個熱門研究方向,深度學習利用多層神經網絡從大量數據中學習特徵和模式,並從中生成高度准確的預測和分類結果。它已被成功應用於語音辨識、圖像識別、自然語言處理等領域,成為當今人工智慧發展的重要推動力。 本論文將深度學習應用於唇語辨識中,利用深度學習的訓練技術來分析人們說話時嘴唇的型態及動作變化來識別語音,以MIRACL-VC1 Dataset為樣本,透過深度學習的技術使用卷積神經網絡(CNN)取唇部特徵值並分別於長短期記憶模型(LSTM)及雙向長短期記憶網絡(BiLSTM)進行訓練並比較其片語準確率,透過適當的數據前處理技術,如時間序列正規化,以及參數的調整,實驗結果皆以ResNet152模型呈現出較好的表現,其中ResNet152與BiLSTM結合後的準確率最高。 ;In recent years, deep learning has emerged as a popular research direction in the field of artificial intelligence. Deep learning leverages multi-layer neural networks to learn features and patterns from vast amounts of data, generating highly accurate predictions and classifications. It has been successfully applied in various domains, including speech recognition, image recognition, natural language processing, and has become a significant driving force in the advancement of artificial intelligence. This paper focuses on applying deep learning to lip reading, utilizing deep learning training techniques to analyze the shape and motion variations of the lips during speech in order to recognize spoken words. The MIRACL-VC1 Dataset is used as the sample dataset. Deep learning techniques, specifically Convolutional Neural Networks (CNN), are employed to extract lip features, followed by training with both Long Short-Term Memory (LSTM) and Bidirectional Long Short-Term Memory (BiLSTM) models. The phrase accuracy of these models is compared. Through appropriate data preprocessing techniques such as time series normalization and parameter adjustment, experimental results demonstrate that the ResNet152 model consistently exhibits superior performance. Particularly, the highest accuracy is achieved when ResNet152 is combined with BiLSTM. In summary, this paper explores the application of deep learning to lip reading, employing deep learning techniques to analyze lip shape and motion during speech for speech recognition. The MIRACL-VC1 Dataset is used, and lip features are extracted using a Convolutional Neural Network (CNN). Training is performed with LSTM and BiLSTM models. By employing suitable data preprocessing techniques and parameter adjustments, experimental results consistently highlight the superior performance of the ResNet152 model, particularly when combined with BiLSTM. |
顯示於類別: | [資訊管理學系碩士在職專班 ] 博碩士論文
|
文件中的檔案:
檔案 |
描述 |
大小 | 格式 | 瀏覽次數 |
index.html | | 0Kb | HTML | 37 | 檢視/開啟 |
|
在NCUIR中所有的資料項目都受到原著作權保護.
|
::: Copyright National Central University. | 國立中央大學圖書館版權所有 | 收藏本站 | 設為首頁 | 最佳瀏覽畫面: 1024*768 | 建站日期:8-24-2009 :::