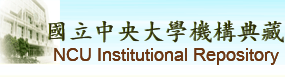 |
English
|
正體中文
|
简体中文
|
全文筆數/總筆數 : 80990/80990 (100%)
造訪人次 : 42685828
線上人數 : 1399
|
|
|
資料載入中.....
|
請使用永久網址來引用或連結此文件:
http://ir.lib.ncu.edu.tw/handle/987654321/93438
|
題名: | 移除弱特徵以抵禦基於行為身份驗證系統上的偽造攻擊;Weak Features Removal Mechanism to Prevent Impersonation Attack on Behavior Biometric System |
作者: | 蘇敬渝;Su, Jin-Yu |
貢獻者: | 資訊工程學系 |
關鍵詞: | 行為生物識別;對抗性攻擊;偽造攻擊;弱特徵;Behavioral Biometrics;Adversarial Attack;Impersonation Attack;Weak Features |
日期: | 2023-08-08 |
上傳時間: | 2024-09-19 17:01:55 (UTC+8) |
出版者: | 國立中央大學 |
摘要: | 隨著科技的成長,人們越來越依賴智慧型手機與網路所帶來的便利性;然而與此發展的同時,一些如行動銀行等包含個人敏感資訊的應用程式造成資料外洩的風險也在持續上升。為此,採用多重的安全措施更能完善系統的安全性。而近年來其中一種備受研究人員關注的身份驗證系統即是基於行為特徵的生物驗證(Behavioral Biometrics),特別是基於操作智慧型手機的行為特徵所建立的身份驗證系統。然而,部分研究也指出,這一類的系統會受到其對抗性攻擊(Adversarial Attack):偽造攻擊(Impersonation Attack),造成系統的穩健性(Robustness)下降。過往,研究團隊曾針對不同的情境,提出識別使用者弱特徵並將其移除之解決方法;然而團隊認為,針對單一使用者與多位攻擊者、多位使用者與多位攻擊者兩種情境的實驗結果仍有進步的空間。因此,本論文將針對單一使用者與多位攻擊者之情境提出識別共同弱特徵(Common Weak Features,CWF)之兩種方法:基本特徵排名法(Baseline Feature Rank, BFR)、反向特徵消去法(Backward Feature Elimination, BFE),並透過移除共同弱特徵的資料擊重新訓練出具有抵禦機制的模型。同時,本論文也將針對多位使用者與多位攻擊者之情境,嘗試透過對CWF投票篩選出總體弱特徵(General Weak Features, GWF),並移除GWF以建立適用所有使用者的通用(General)抵禦模型。除此之外,本論文也考量到使用者間的多樣型,提出了另一種基於預測試(Pretesting)與分群(Clustering)架構的方法,並在初步實驗透過使用者的共同弱特徵衡量使用者間的相似度(Similarity),並為使用者進行分群,以證實該架構的可行性,為研究團隊提供了一個基礎架構,供其進一步建立和改進。;With the development of technology, people are increasingly relying on the convenience brought by smart phones and the Internet. However, some applications such as mobile banking that contain sensitive personal information cause data leakage risks is continuing to rise at the same time. For this reason, multiple security measures are implemented to have the system as secure as possible. In recent years, one of the authentication systems that getting more attention from researchers is behavioral biometrics, especially the touch interaction of operating smart phones. However, some studies also pointed out that those systems are attacked by its adversarial attack: impersonation attack, resulting in a decrease in the robustness of the system. Our research team has proposed different solutions to identify weak features in different scenarios of users and remove them for rebuild the defense model in the past, but the results of some scenarios still have room for improvement. Therefore, this thesis will propose two methods: Baseline Feature Rank (BFR) and Backward Feature Elimination (BFE) for identifying Common Weak Features (CWF) in the scenario of 1 user versus multiple attackers. The defense model will be trained by data that remove with CWF. Additionally, this thesis also aims to address scenarios involving multiple users and multiple attackers. It attempts to identify the General Weak Features (GWF) by applying voting to the CWF and subsequently removing the GWF to build the general defense model applicable to all users. Furthermore, considering the diversity among users, this thesis also proposes an alternative approach based on the pretesting and clustering framework. In our initial experiments, the similarity between users is measured through their CWF, and clustering is performed to validate the feasibility of this framework. This provides a foundational structure for research teams to further develop and improve upon. |
顯示於類別: | [資訊工程研究所] 博碩士論文
|
文件中的檔案:
檔案 |
描述 |
大小 | 格式 | 瀏覽次數 |
index.html | | 0Kb | HTML | 25 | 檢視/開啟 |
|
在NCUIR中所有的資料項目都受到原著作權保護.
|
::: Copyright National Central University. | 國立中央大學圖書館版權所有 | 收藏本站 | 設為首頁 | 最佳瀏覽畫面: 1024*768 | 建站日期:8-24-2009 :::